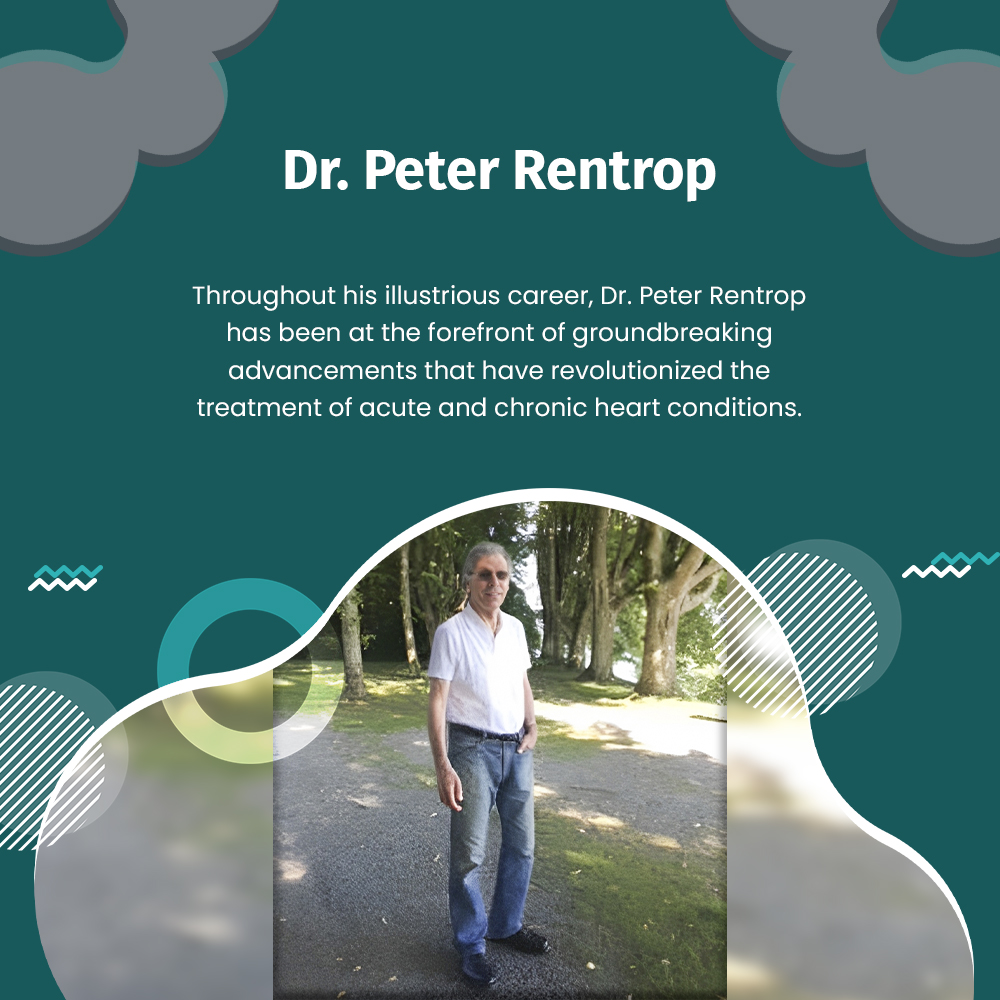
Advances in artificial intelligence (AI) are redefining the landscape of medicine. Among the most significant breakthroughs is the use of AI in diagnostics, which allows for disease prediction with unmatched accuracy. By leveraging vast datasets and cutting-edge algorithms, AI is improving early detection and treatment strategies. As this technology continues to evolve, its implications for healthcare are profound, promising better outcomes and more personalized patient care.
While the concept of AI in medicine may once have seemed futuristic, its practical applications now make a tangible difference in diagnosing and predicting diseases.
How AI Analyzes Data to Improve Diagnostics
AI relies on data—lots of it. Through sophisticated machine learning algorithms, it processes and analyzes often too subtle patterns for human detection. This enables AI to identify potential health issues long before symptoms manifest. For example, algorithms trained on imaging data can detect early-stage cancer in scans, offering a chance for timely intervention.
Additionally, AI systems incorporate data from diverse sources, such as electronic health records (EHRs), wearable devices, and genetic profiles. This holistic approach allows AI to account for various factors contributing to disease development. Consequently, healthcare providers can deliver more precise diagnoses and tailor treatment plans to individual patients. Importantly, this data-driven approach enhances diagnostic accuracy and minimizes the risk of misdiagnosis.
Beyond improving accuracy, AI significantly speeds up the diagnostic process. In traditional settings, interpreting medical images or test results could take hours or even days. However, AI systems can analyze complex data in seconds, helping doctors make quicker decisions. This efficiency can be life-saving, especially in emergency or critical care scenarios.
Transforming Disease Prediction Through Machine Learning
Machine learning plays a central role in AI-driven diagnostics. These systems analyze historical data to identify risk factors and predict potential health outcomes. For instance, AI algorithms can assess a person’s likelihood of developing chronic diseases such as diabetes or heart disease by analyzing lifestyle habits, genetic predispositions, and medical history.
Moreover, predictive models are growing increasingly sophisticated. With each dataset they process, machine learning algorithms refine their ability to foresee patterns, making predictions more reliable. Hospitals and clinics worldwide are already implementing these systems to identify at-risk patients, enabling preventative care and reducing the overall burden on healthcare systems.
This predictive capability is particularly impactful in combating global health crises. During the COVID-19 pandemic, AI systems analyzed large datasets to predict outbreak trends, helping governments and health organizations allocate resources efficiently. Similarly, AI predicts how specific cancers might progress in oncology, allowing oncologists to plan more effective treatment protocols.
While the results are promising, applying machine learning in diagnostics requires careful oversight. Ensuring these systems are transparent, unbiased, and ethical is essential to maximizing their potential and maintaining healthcare trust.
Integrating AI Diagnostics Into Clinical Practice
Integrating AI diagnostics into routine medical practice involves bridging the gap between technology and human expertise. While AI excels at data analysis, physicians provide the clinical context to ensure accurate diagnoses. Collaboration between the two is crucial to delivering the best outcomes for patients.
Similarly, primary care physicians are beginning to use AI-powered platforms to streamline patient care. For example, chatbots equipped with AI can handle preliminary consultations by collecting symptoms and medical histories. This allows doctors to allocate their time more efficiently, focusing on patients who require immediate attention.
Despite these advantages, challenges remain. Physicians must be trained to understand and utilize AI tools effectively. Moreover, ensuring the interoperability of AI systems with existing medical software is critical for seamless adoption. While these hurdles require attention, the growing evidence supporting AI diagnostics underscores its transformative potential.
Ethical Considerations and Challenges in AI-Driven Healthcare
While AI promises remarkable advancements, its integration into healthcare also raises important ethical and logistical questions. Data privacy, algorithm bias, and accountability are critical concerns that must be addressed to ensure ethical implementation.
Data privacy is paramount in AI diagnostics. Since AI systems rely on vast amounts of personal health data, safeguarding this information is crucial. Strong encryption protocols and adherence to regulations like HIPAA are essential to protecting patient confidentiality. Additionally, healthcare providers must remain transparent about how AI systems use and store data, fostering patient trust.
Algorithm bias is another pressing issue. If training datasets do not represent diverse populations, AI models may produce skewed results, disproportionately affecting certain groups. Addressing this requires inclusive datasets and rigorous testing to ensure equitable outcomes across demographics.
The Future of AI Diagnostics in Healthcare
Looking ahead, the potential for AI diagnostics to revolutionize healthcare is boundless. With natural language processing and computational power advancements, AI systems are expected to become even more accurate and versatile. Additionally, the proliferation of wearable health devices will generate more real-time data, enhancing AI’s predictive capabilities.
Furthermore, the global reach of AI diagnostics is expanding. In regions with limited access to healthcare, AI-powered tools are filling critical gaps by providing remote diagnostic capabilities. This democratization of healthcare has the potential to save countless lives, especially in underserved areas.